A trust network is constituted by a social grid in which touch points represent the trust relationship between two nodes in the network. These trust systems are directly influenced by bias; whereby the “bias of a node measures the trustworthiness of a node” (A Framework of Algorithms: Computing the Bias and Prestige of Nodes in Trust Networks, Rong-Hua Li).
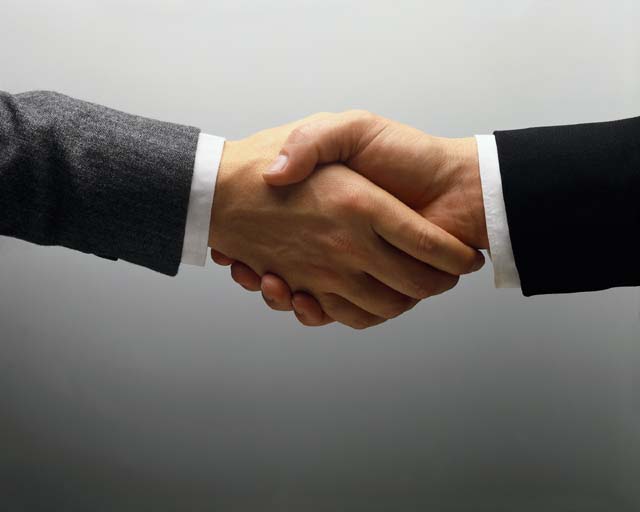
Making trust connections.
Essentially, trust networks are founded on the basis that the greater bias of a node, the lower trustworthiness of the node, and the similarly, the greater the reputation of the node, the higher importance of the node in that network. We can bring this concept into the real world by saying simply, the greater a person’s bias, the less trustworthy they will be on a grander scale; and the larger a person’s respect or reputation, the more important they are to that specific trust social network.
Trust networks house many different applications: we can find the trusted nodes in a specific network, forecast the trust score of other nodes and systematically vouch for a trusted organisation or online social and ethical structure.
Richters and Peixoto examined the connection between “an implicit trust network” and trust broadcasting on social networks. This related to the idea related above with regards to the influence of bias, transparency and “prestige”. They ran into a few challenges with their definition and measurement of “reasonable bias” that would encapsulate the bias of consumers’ attitudes, and the most efficient handling of negative trust scores in signed trust networks. Ultimately, they struggled to produce a strong, faultless algorithm which could thwart attack from some confrontational users. However, in the last few years, sources such as PageRank have designed algorithms that have enabled users to test and allow for negative touch points in the case of signed networks.
Mishra and Bhattacharya designed and put forward an algorithm, referred to as MB, that tackled the measurement of “prestige and bias” of nodes in a trust social web. Specifically designed for signed trust networks, MB could also be used for unsigned networks also, which made it even more desirable.
Adrian Miles alludes to the rapid direction we are taking towards new media forms and growing social networks. Network literacy is an evolving social form which dictates whether an advertisement of piece of collaborative content is recognised and understood by its audience. Whether a person can understand the web networks occurring today underpins their understanding of forms such as native advertising, online ethics and disclosure.
He describes this ability as “being comfortable with change and flow as the day to day conditions of knowledge production and dissemination, and recognising that all of this may change…”
He goes on to explain that, “What underlies [this] change, however, are the principles of distributed content production and sharing, folksonomies, trust networks and having access to skills that let you collate and build with these varieties of content and knowledge… Network literacy means recognising that there are no longer canonical sources and having the skills to find what it is you think you want, of being able to judge it, and then of being able to incorporate this, in turn, into your knowledge flows.”
References:
Huttenlocher, Kleinberg, Leskovec, 2010, ‘Signed Networks in Social Media’, Machine Learning and Web Interactions, April, pp. 1361 – 1369.
Chan, Cho, J & K 2013, ‘Building Trust-Based Sustainable Networks’, IEEE Technology and Society Magazine, December, Issue 1, pp. 33 – 38.
Cheng, Huang, Li, 2012, ‘A Framework of Algorithms: Computing the Bias and Prestige of Nodes in Trust Networks’, Department of Systems Engineering & Engineering Management The Chinese University of Hong Kong, October, Issue 1, pp. 1 – 7.
Di Cagno, Sciubba, D & E 2010, ‘Trust, trustworthiness and social networks: Playing a trust game when networks are formed in the lab’, Journal of Economic Behavior & Organization, April, pp. 1 – 11.
Miles, Adrian. “Network Literacy: The New Path to Knowledge.” Screen Education Autumn.45 (2007): 24–30.